A Phased Approach to AI Adoption in Network Operations & Maintenance
To meet the goals of network quality, customer experience and cost containment, operators will need to embrace even more intelligent forms of network maintenance.
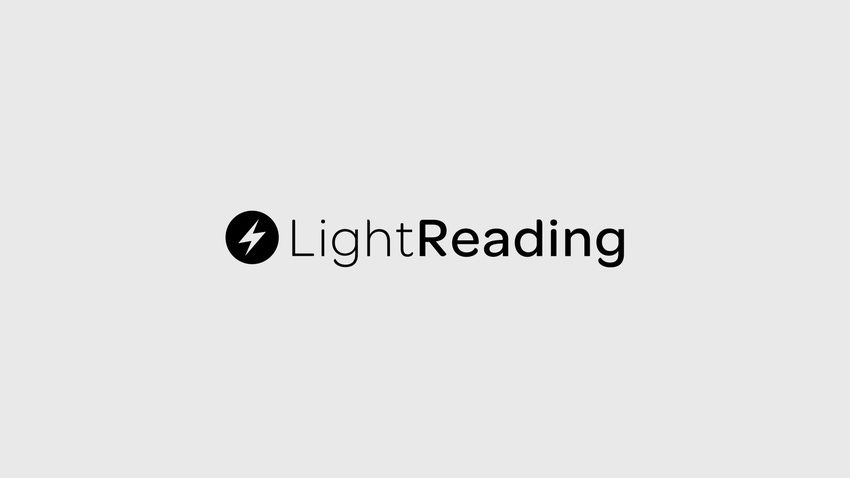
Here's a story that will seem familiar to many operators across the world: Not so long ago, China Mobile was struggling to simultaneously ensure network quality and improve customer experience while at the same time reduce its operational costs.
A key enabler to achieve these goals was its shift from reactive maintenance not just to preventative maintenance, but increasingly to predictive maintenance. China Mobile divided its intelligent operations and maintenance (O&M) evolution into five phases and gradually increased automation and its reliance on Artificial Intelligence (AI).
In the first phase, AI is used solely to identify the warning signs of potential faults.
In the second phase, the intelligent O&M system used AI to indicate the likely cause of the predicted fault.
In the third phase, the system predicted when the fault is likely to occur and with what probability; this provides the basis for engineers to take the necessary countermeasures.
In the fourth phase, the AI becomes more autonomous, determining which measures should be taken, although engineers are still required to make the actual changes.
In the fifth and final phase, still under development, the AI operates in an automated closed loop, carrying out network repair without the need for human intervention (self-healing).
In parallel with this phased approach to the adoption of AI in O&M, China Mobile used four strategic approaches to ensure the robustness of its network.
The first strategic approach was called "Real-time Risk Prediction for VoLTE." Traditionally, faults on VoLTE services have only been identifiable after-the-fact through alarms or (even worse) user complaints. Instead, China Mobile now collects a time series of data (KPIs, error codes, etc.) from the live network and analyzes it for anomalies. Algorithms are applied to the data in order to build fault prediction models for different service types (such as VoLTE). Data from the live network is compared with the models in real time in order to identify potential faults. China Mobile has found that this intelligent risk prediction can identify network faults several hours in advance.
The second strategic approach is "Automatic Fault Diagnosis." Again, various network data (traffic statistics, alarms, operation logs) is collected along with Call History Records (CHRs) and alarms/IP data generated by faults. Correlations are then identified across nine dimensions between faults and factors such as telephone number, terminal, cell, and Network Element (NE). These correlations are then converted into rules (using the open source service rule engine Drools) that can be executed by a maintenance IT platform. China Mobile has found that taking advantage of machine intelligence in this way greatly improves the efficiency of analyzing the huge amount of alarms and log data that are generated each day.
The third strategic approach is "Network Cutover Assurance." To ensure successful cutover (e.g. replacing a switch in a live network), traditionally a cutover project team would formulate detailed implementation plans. However, cutover blunders still frequently occur. To avoid this, China Mobile sought to add greater intelligence across the three phases of the cutover: operation, verification, and on-duty support. In the operation phase, intelligent risk detection is used to implement monitoring policies that identify errors and alert staff to correct them before services are impacted. In the verification phase, there is an automatic analysis of alarms, logs, and dialing test/CHR (call history records). During the on-duty support phase, user complaints are monitored in real time and the likely causes of these complaints are automatically identified.
The fourth and final strategic approach is "Online, Intelligent Evaluation." Traditional network inspection methods are inefficient and require a high level of expertise. With intelligent evaluation systems, the operator can more easily check logs, device configurations, and the trends and real-time status of device software and hardware. According to Huawei, when routine maintenance is defined as rules, real-time data collection and intelligent analysis can improve the accuracy of network risk evaluation by 90%.
With new technologies such as 5G and SDN/NFV, CSPs need more intelligent network maintenance that takes advantage of machine learning and big data analysis. Predictive maintenance is one of a series of steps in the evolution of network management. To meet the goals of network quality, customer experience and cost containment, operators will need to embrace even more intelligent forms of network maintenance that enable the automated identification of problems, next-best-action recommendations, and ultimately self-healing.
This blog is sponsored by Huawei.
— James Crawshaw, Senior Analyst, Heavy Reading
About the Author(s)
You May Also Like