Orange isn't building its own AI foundation model – here's why
Orange is focusing on using methods like RAG and fine-tuning to make better use of existing LLMs, rather than undertaking the highly complex task of building its own foundation model, its chief AI officer says.
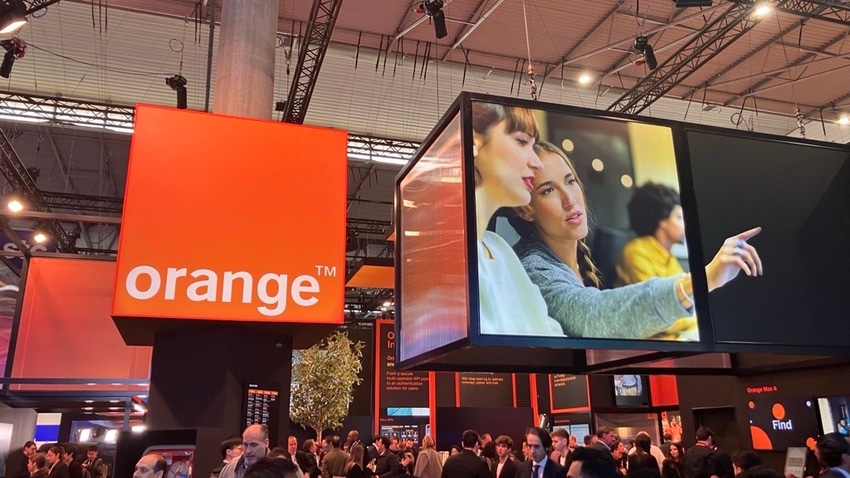
There has been a flurry of interest in generative AI (GenAI) from telcos, each of which has taken its own nuanced approach to the idea of building its own large language models (LLMs). While Vodafone seems to dismiss the idea and Verizon appears content to build on existing foundation models, Deutsche Telekom and SK Telecom announced last year they will develop telco-specific LLMs. Orange, meanwhile, doesn't currently see the need to build a foundation model, its chief AI officer Steve Jarrett has recently told Light Reading.
Jarrett said the company is currently content with using existing models and adapting them to its needs using two main approaches. The first one is retrieval-augmented generation (RAG), where a detailed source of information is passed to the model together with the prompt to augment its response.
He said this allows the company to experiment with different prompts easily, adding that existing methodologies can be used to assess the results. "That is a very, very easy way to dynamically test different models, different styles of structuring the RAG and the prompts. And […] that solves the majority of our needs today," he elaborated.
At the same time, Jarrett admitted that the downside of RAG is that it may require a lot of data to be passed along with the prompt, making more complex tasks slow and expensive. In such cases, he argued, fine-tuning is a more appropriate approach.
Distilling models
In this case, he explained, "you take the information that you would have used in the RAG for […] a huge problem area. And you make a new version of the underlying model that embeds all that information." Another related option is to distill the model.
This involves not just structuring the output of the model, but downsizing it, "like you're distilling fruit into alcohol," Jarrett said, adding "there are techniques to actually chop the model down into a much smaller model that runs much faster."
This approach is, however, highly challenging. "Even my most expert people frequently make mistakes," he admitted, saying: "It's not simple, and the state of the art of the tools to fine tune are changing every single day." At the same time, he noted that these tools are improving constantly and, as a result, he expects fine-tuning to get easier over time.
He pointed out that building a foundation model from scratch would be an even more complex task, which the company currently doesn't see a reason to embark on. Nevertheless, he stressed that it's impossible to predict how things will evolve in the future.
Complexity budget
One possibility is that big foundational models will eventually absorb so much information that the need for RAG and other tools will diminish. In this scenario, Orange may never have to create its own foundation model, Jarrett said, "as long as we have the ability to distill and fine tune models, where we need to, to make the model small enough to run faster and cheaper and so on."
He added: "I think it's a very open question in the industry. In the end, will we have a handful of massive models, and everyone's doing 99% RAG and prompt engineering, or are there going to be millions of distilled and fine-tuned models?"
One factor that may determine where things will go in the future is what Jarrett calls the complexity budget. This is a concept that conveys how much computing was needed from start to finish to produce an answer.
While a very large model may be more intensive to train in the beginning, there may be less computing required for RAG and fine-tuning. "The other approach is you have a large language model that also obviously took a lot of training, but then you do a ton more compute to fine tune and distill the model so that your model is much smaller," he added.
Apart from cost, there is also an environmental concern. While hyperscalers tend to perform relatively well in terms of using clean energy, and Jarrett claimed that Orange is "fairly green as a company," he added that the carbon intensity of the energy used for on-premises GPU clusters tends to vary in the industry.
Right tool for the job
The uncertainty surrounding GenAI's future evolution is one of the reasons why Orange is taking a measured approach to the technology, with Jarrett stressing it is not a tool that's suited to every job. "You don't want to use the large language model sledge hammer to hit every nail," he said.
"I think, fairly uniquely compared to most other telco operators, we actually have the ability, the skill inside of Orange to help make these decisions about what tool to use when. So we prefer to use a statistical method or basic machine learning to solve problems because those results are more […] explainable. They're usually cheaper, and they're usually less impactful on the environment," he added.
In fact, Jarrett says one of the things Orange is investigating at the moment is how to use multiple AI models together to solve problems. The notion, he added, is called agents, and refers to a high-level abstraction of a problem, such as asking how the network in France is working on a given day. This, he said, will enable the company to solve complex problems more dynamically.
In the meantime, the company is making a range of GenAI models available to its employees, including ChatGPT, DALL-E and Mistral. To do so, it has built a solution that Jarrett says provides a "secure, European-resident version of leading AI models that we make available to the entire company."
Improving customer service
Jarrett says this is a more controlled and safer way for employees to use models than if they were accessed directly. The solution also notifies the employee of the cost of running a specific model to answer a question. Available for several months, it has so far been used by 12% of employees.
Orange has already deployed GenAI in many countries within its customer service solutions to predict what the most appealing offer may be to an individual customer, Jarrett said, adding "what we're trialling right now is can generative AI help us to customize and personalize the text of that offer? Does that make the offer incrementally more appealing?"
Another potential use case is in transcribing a conversation with a customer care agent in real time, using generative AI to create prompts. The tool is still in development but could help new recruits to improve faster, raising employee and customer satisfaction, said Jarrett.
While Orange doesn't currently use GenAI for any use cases in the network, some are under development, although few details are being shared at this stage. One use case involves predicting when batteries at cell sites may need replacing.
Jarrett admits, however, that GenAI is still facing a number of challenges, such as hallucinations. "In a scenario where the outputs have to be correct 100% of the time, we're not going to use generative AI for that today, because [it's] not correct 100% of the time," he said.
Dealing with hallucinations
Yet it can be applied in areas that are less sensitive. "For example, if for internal use you want to have a summary of an enormous transcript of a long meeting that you missed, it's okay if the model hallucinates a little bit," he added.
Hallucinations cannot be stopped entirely and will likely continue to be a problem for some time, said Jarrett. But he believes RAG and fine-tuning could mitigate the issue to some extent.
"The majority of the time, if we're good at prompt engineering and we're good at passing the appropriate information with the response, the model generates very, very useful, relevant answers," Jarrett said about the results achieved with RAG.
The availability and quality of data is another issue that is often discussed, and also one that Orange is trying to address. Using data historically kept in separate silos has been difficult, said Jarrett. "[The] availability of the data from the marketing team to be able to run a campaign on where was our network relatively strong, for example – those use cases were either impossible, or took many, many, many months of manual meetings and collaboration."
As a result, the company is trying to create a marketplace where data is made widely available inside each country and appropriately labeled. Orange calls this approach data democracy.
UPDATE: The story has been updated to reflect that DALL-E, not Dolly, is among the GenAI models Orange has made available to employees.
About the Author(s)
You May Also Like