SDN + AI: A Powerful Combo for Better Networks
AT&T and Level 3 execs explain how AI and machine learning are being built into network platforms that deliver more reliability and security.
August 29, 2016
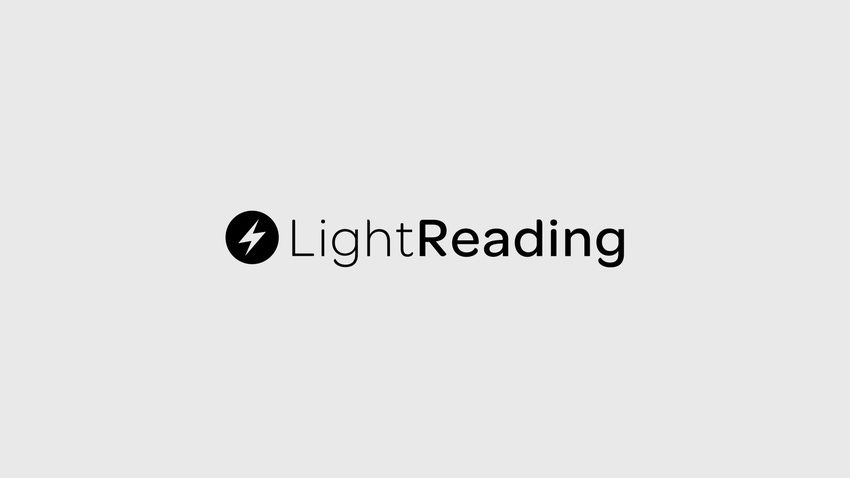
The combination of software-defined networking and machine learning/artificial intelligence is becoming a powerful tool for making networks more reliable and secure.
And while not everyone is willing to talk about their activities yet -- CenturyLink Inc. (NYSE: CTL) and Verizon Communications Inc. (NYSE: VZ) declined interview requests on this topic -- a peek inside what is happening at AT&T Inc. (NYSE: T) and Level 3 Communications Inc. (NYSE: LVLT) offers a clear view of what's possible. In this first of two stories, executives at those companies share how machine learning and AI are being built into their networks today.
As Mazin Gilbert, AVP of Intelligent Services at AT&T Labs, explains, artificial intelligence and machine learning are hardly new concepts, nor is the idea of using these tools to improve network performance and security. There was talk about that as far back as the 1980s, he says.
But AI and machine learning "are engines that feed on data, like a car engine feeds on gasoline," Gilbert explains. "They require extensive computation to be able to make recommendations and decisions. Now we are at the era where we can do that and we can do it cost effectively. We can do it in a way that impacts the service and the application and the experience in real time, live as that communication is happening."
At Level 3, "we are passionate about this and have major investment going into" artificial intelligence and machine learning, says Travis Ewert, VP of network software development. Internally, the company has branded these efforts "See, Know and Act," for the three stages of collecting data, analyzing it and taking action on that analysis.
Both companies are combining their human intelligence, developed over decades of experience, with machine learning and AI in ways that take advantage of both. The critical piece of the latter is the much-coveted ability to rapidly analyze massive volumes of information to detect patterns, trigger warnings and find anomalies that can help network operators predict a range of network issues before they impact customers.
"Human experts are typically excellent at understanding context," Gilbert says. "We also are very good at understanding logic and we are very good at making reasonable and informative decisions. Those are weak areas of AI systems. We are taking the key differentiator of human experts and key differentiators of AI systems and bringing them together to provide us the security, reliability and best experience in our network."
Staggering numbers
To frame the "massive data" discussion, consider these numbers from Level 3: According to Ewert, Level 3 is collecting threat information on about 50 billion events a day across its global network. It is collecting tens of millions of NetFlow messages -- those generated by Cisco routers -- daily as well. On its global content delivery network infrastructure, Level 3 collects about 2 million logs a second, while on its data network, it gets about 3 million messages a second on network and service performance, tracking things like latency, loss and jitter in both passive and active ways.
All of that data goes into four massive data lakes that Level 3 maintains globally.
"What is kind of cool now is that we are grabbing so much data that all of a sudden, our lakes have become an ocean," Ewert says. "And that's just the 'See' piece of the branding statement, where it starts: Can you collect all of that data at scale?"
Want to know more about carrier SDN strategies? Check out our dedicated SDN content channel here on Light Reading.
AT&T also collects centralized data, for analysis, but Gilbert is more excited about discussing the data collection at the very edge of AT&T's network, made possible by the software-defined networking that allows the distribution of network intelligence to that edge.
"We can examine data at the end of the flow, which we have traditionally done," he says. "By putting the intelligence at the edge, and being able to detect that [issue or anomaly] fast enough and address that fast enough -- not ten or 20 minutes later -- that could be the tremendous difference between a disaster and having the best experience. It becomes a matter of how fast and how efficiently and how accurately you are able to address those issues."
Being able to analyze data in motion, starting at the edge, is what makes rapid response possible, Ewert says, and also enables a much higher degree of security.
"That is where things like string analytics, advanced algorithms, machine learning -- all of those things come into play," he comments. "The key to this one is how do you draw knowledge from this data that is in motion. We have built in some streaming analytics where we can literally be running analytics or advanced algorithms before the data goes to long-term data store, so we can look for triggers or detection points or 'if-then' statements and that could be for network or service performance."
So when latency is detected, or a certain level of utilization, an automatic response is triggered that mitigates the problem, or when a certain alignment of data matches a suspected threat profile, the network can quarantine or block traffic for security mitigation purposes.
"We have literally got algorithms on tens of thousands of flows," Ewert says. "And that gives us the ability to look at all those streams of data to be able to baseline a known state." The current traffic patterns can then be compared to that baseline, in detecting anomalies that might trigger a response, and those baseline comparisons can be done based on time of day or day of the week, or multiple other factors.
A new network platform
Some of what AT&T is doing today in its software-centric transformation of its network was done previously in individual applications or services or for specific customers, Gilbert says. But the move to SDN is enabling AT&T to build machine learning and artificial intelligence into its network platform, so they become tools on which all other services and applications are built.
"It becomes core to our network," he says. "Our network is not just serving big customers or high-valued customers only; it is serving everybody. We are going from an application-centric solution to a platform-centric network solution that is really supporting everybody and all applications."
Artificial intelligence is being used to monitor the behavior of the virtual machines and applications running in AT&T's SDN, so that when any issues or degradations are noticed, the decision can be quickly made on how to handle that issue. "That is where we apply our traditional intelligence to decide whether we move the traffic to another machine without the customer even knowing or without being impacted, or do we reboot the machine during a downtime, or is there another solution," Gilbert says. "That is what we are doing today to help us build a more reliable network."
Tomorrow: What lies ahead
— Carol Wilson, Editor-at-Large, Light Reading
You May Also Like