New Heavy Reading report highlights the growing role of AI in networking operational use cases
In the current Heavy Reading report, “Intelligent Data-Driven Operations with AIOps” we focus on artificial intelligence for IT operations, building on the subject of how communications service providers (CSPs) can simplify network management by automating multi-layer operations – which we examined in the earlier white paper, Simplifying Operations with Multi-Layer Network Control. #Sponsored
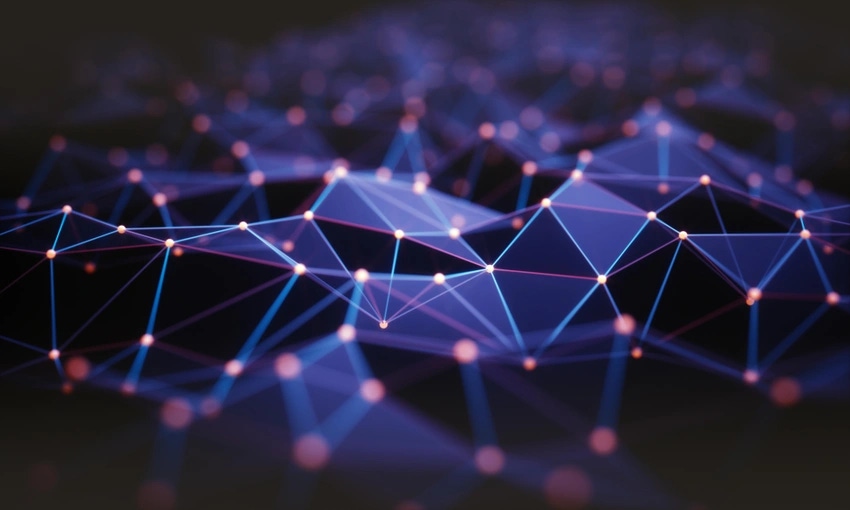
In the current Heavy Reading report, “Intelligent Data-Driven Operations with AIOps” we focus on artificial intelligence for IT operations, building on the subject of how communications service providers (CSPs) can simplify network management by automating multi-layer operations – which we examined in the earlier white paper, Simplifying Operations with Multi-Layer Network Control. CSPs are now looking to use AIOps to broaden the scope, ease of use, and effectiveness of network management in all stages of the network lifecycle.
CSPs are leveraging a combination of AI/ML, multi-layer management, and the cloud to underpin their AIOps strategy, as shown in Figure 1. Heavy Reading sees specific current use cases benefiting most from AIOps over the next two years, providing the best ROI for the CSPs. These use cases are predominantly in the network operations and management space, as suggested by Omdia’s examination of telecom AI contracts a year ago. More specifically, most are performance optimization use cases, which improve routes, channels, and bandwidth/spectrum utilization while supporting SLA parameters and route diversity requirements.
Figure 1: CSPs are looking to improve efficiency and cut hardware costs with a shift to cloud
Source: Omdia, Service Provider Digital Transformation and Cloud Strategies Survey Report – 2023
Key AI/Ops use cases are described below. Multi-layer management capability is essential to all of these use cases for merged Layer 0–3 visibility and control across optical and IP layers.
Proactive maintenance: AI/ML is used to predict maintenance issues, identify the root cause, and ultimately resolve the issue without operator intervention. Verifying fiber health is an example of proactive maintenance. It usually relies on an optical time domain reflectometer (OTDR), a device that tests the integrity of a fiber cable and is used for the building, certifying, maintaining, and troubleshooting of fiber optic systems. Current OTDR capability is embedded into photonic line cards, making frequent measurement possible. AI/ML modules leverage this historical and current OTDR data to enable proactive maintenance apps.
Multi-layer capacity forecasting: By analyzing historical and trend data from Layers 0–3 AI can be used to predict future demand. In delivering a prediction of future capacity, such forecasting also considers the impact of changes to the individual layers (IP, Ethernet, or optical) and the cost impact of the capacity recommendations.
Congestion alleviation: This uses ML to identify patterns and relationships in traffic data to move traffic to avoid congestion or to identify distributed denial-of-service (DDoS) attack traffic and drop it.
Signal-to-noise ratio optimization: AI/ML in combination with analytics can be used to monitor and control the signal-to-noise ratio (SNR) margin. Today’s coherent modems generate a massive amount of data, but without AI/ML, it is difficult to leverage the data in a timely and holistic manner. If CSPs can increase the SNR on their optical infrastructure, they can optimize costly fiber transport and significantly lower the overall cost per bit.
Spectrum defragmentation: Spectrum becomes fragmented when isolated, non-aligned, and non-contiguous frequency slots accumulate, and spectrum cannot be allocated to new connections. Defragmentation of the spectrum results in better use of the fiber and the freeing up of stranded capacity. This use case is computationally intensive and relies on AI/ML to determine the optimal channel assignments to free up the most spectrum.
Digital Twins: Digital twining creates a virtual representation of a physical entity, operational process, or set of data in order to simulate, capture real-time performance data, and predict and optimize. This gives CSPs the tools they need to answer questions such as, “Can I complete a network modification in a maintenance window?” Digital twinning also enables CSPs to test software upgrades (e.g., to routers) by first providing scripts that can be validated before installing the upgrade on the production network. For CSPs, a digital twin provides a replica of the physical network that exists in the digital space throughout the network lifecycle. A digital twin continuously learns and updates itself from multiple sources to show its near real-time status and features, working conditions, or position. It also integrates historical data from past machine usage into its digital model.
CSPs consistently cite AIOps and the technologies that make up an AIOps solution—AL, ML, multicloud, cloud native migration, etc.—as critical parts of their digital transformation strategy. They emphasize that the transition to AIOps cannot take place overnight. However, they recognize AIOps as a solution for dealing with relentless network expansion and see it is imperative for managing the network going forward.
This blog post is sponsored by Ciena.
About the Author(s)
You May Also Like