Evolving into AI-native networks
AI-native networks will emerge at the intersection of new working practices, successful collaboration and high confidence.
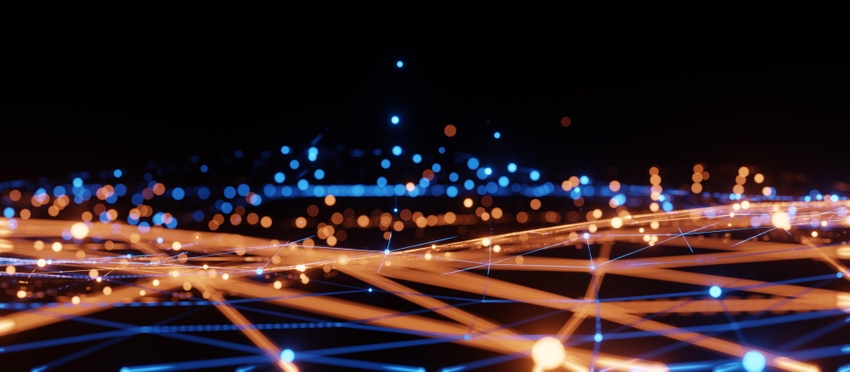
Mobile network operators face a continual challenge: How can they improve performance and efficiency and drive profitability? The real-time nature of 5G services puts the focus on streamlined operations and artificial intelligence (AI)-assisted autonomy. Mobile networks are critical environments, and security or fault outages come with high costs and reputational penalties. Hence, operators must tread carefully toward AI in their networks.
Predictive AI and generative AI (GenAI) have experienced a surge of interest, but also hype, from industry stakeholders. Operators cannot ignore the path to AI-native networks and the potential role that predictive and GenAI will play in the future. They are already developing and piloting new AI network use cases to speed task execution, grow new service offerings, transform efficiency and deliver exceptional customer experience.
Defining a plan to remove humans from the decision network loop is an operator objective. AI advocates agree that this evolution will not happen overnight. Instead, it will progress in stages from basic, overlaid AI operations within smaller zones to larger automated processes. Ultimately, AI will become an inherent capability of the network.
The journey: Guardrails and training wheels
Operators are optimistic about the potential of AI to improve network performance and economics. They are also mindful that the new technology will require guardrails and support to reach full autonomy.
"This is a journey; it's a rather fundamental shift. You have to address culture, collaboration and competency over some time," said Terje Jensen, a senior VP at Telenor Group, during a recent Light Reading symposium on AI.
Embedding AI to enhance business processes, services and operations requires trust, aptitude and accuracy. Operators' infrastructure and data are accountable to regulators, national laws and customers. Accordingly, AI quality-assisted modes with human feedback will form over time, allowing trust and confidence in the algorithms to build. Re-examining the following aspects is essential:
Culture: Network operations teams and skill sets must evolve to support AI-native, and AI technology must be explainable and transparent. Both traits are essential to technology adoption and to allowing ops teams to understand why an AI model is making a decision, as well as any possible limitations. AI must also be ethical and maintain, at a minimum, current operational guidelines and legal frameworks, including accountability, data integrity, contextual understanding and safety, equality, etc.
Industry and collaboration: Forging ecosystem agreement and standardization is vital to harmonizing interface, process and data definitions and preventing proprietary solutions or lock-in. 3GPP standardizes AI across management and orchestration, RAN and core domains with functions such as management data analytics (MDA), RAN intelligence and network data analytics function (NWDAF). Further work continues to define a machine learning (ML) entity to manage and secure inter-vendor model use and security risks.
Accuracy: Assuring that the precision of real-time processes and their output are met will be iterative and require ongoing assistance to build quality and confidence (e.g., open-loop AI). Ensuring data quality is also essential, and many operators are already amalgamating data silos within their operations, along with continued data quality and curation checks.
A surge of AI use cases to try
AI is not new; intelligent voice recognition systems have supported operators for many years. They are now looking for more sophisticated AI use cases to bring benefits to business, service and network operations. As a result, developments and trials are underway:
Energy efficiency
Deutsche Telekom is one of the many vendors trialing RAN intelligence control (RIC) to optimize a disaggregated RAN. In a collaborative trial integrating Juniper and VMware RICs, as well as VIAVI Solutions and AirHop rApps, the partners demonstrated energy savings dynamic multi-carrier management (ESMC) in the RAN using AI/ML to determine the best time for sleep modes based on capacity cells.
Security, fraud and root cause analysis (RCA)
Detecting fraudulent language patterns (e.g., asking for credit card numbers or account details) is another high potential AI use case for analyzing calls in real time. If fraud is detected, the system will interrupt the call and alert the user that it may be fraudulent. Microsoft Azure is currently testing its Operator Call Protection with BT Group.
The RCA of network and security issues is critical. Fujitsu is exploring GenAI to assist NetOps teams with RCA via network knowledge extraction and using a large language model (LLM). Teams receive evidence-based recommendations with a confidence level, which they can assess to increase the system's accuracy.
Customer service and care
Customer contact centers are typically large teams. AI-powered assistance allows human agents to focus on complex or specialized tasks. Zain KSA is collaborating with Google Cloud and its Contact Center AI (CCAI) solution to trial Arabic bots for enhanced customer experience.
AI-native networks will emerge at the intersection of new working practices, successful collaboration and high confidence. A multiphased approach to developing these networks is required to further prove their value.
About the Author(s)
You May Also Like